A group of automated vehicle technology companies have teamed up to create a pre-integrated hardware and software platform for auto manufacturers. They claim they can build and deploy continuously updated AI machine learning models 10 times faster, with data reductions as high as 98% and accuracy greater than 99%.
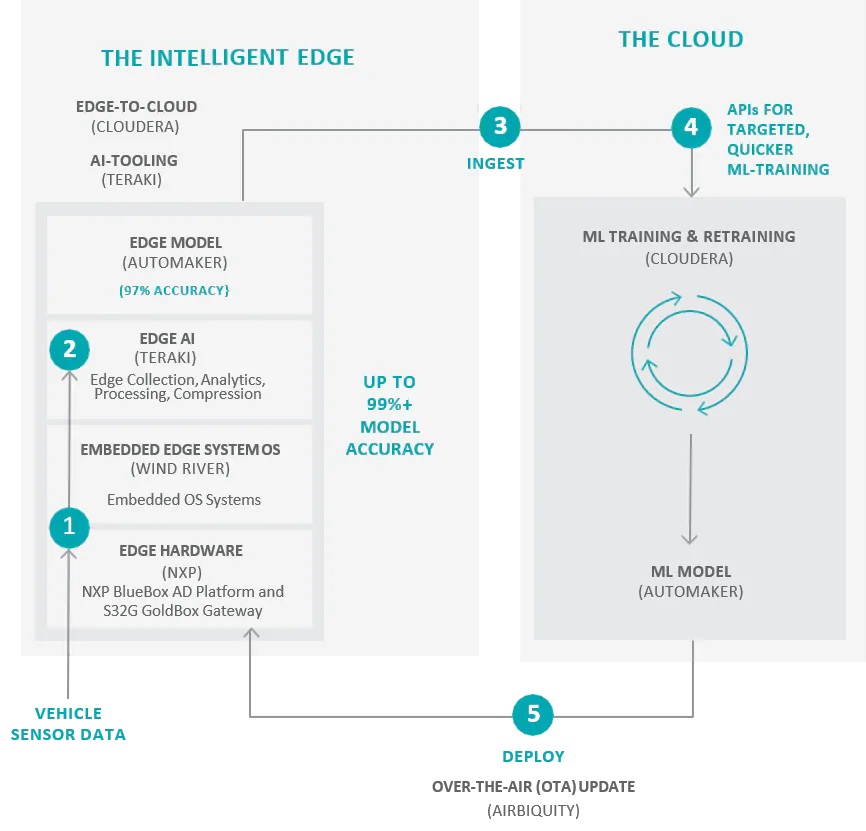
Credit: NXP
The “vehicle to cloud” system ingests new sensor data from different driving scenarios and processes it with in-vehicle and edge computing devices before sending it to the cloud. There, AI software trains/retrains ML models based on the new data and transmits updates back to the vehicle over the air (OTA).
The companies—Airbiquity, Cloudera, NXP, Teraki and Wind River—say they’re addressing common challenges the AV industry faces to leverage the burgeoning reams of data collected by increasingly sophisticated vehicles sensors for better autonomy. Automakers can evaluate and introduce the system into vehicle design and production cycles, and scale it from Advanced Driver Assistance System (ADAS) applications to further autonomous driving capabilities.
The collaboration, project leaders say, is different than others. Dream-team partnerships have grabbed headlines—this year alone, Microsoft has announced individual AV ventures with Volkswagen, General Motors and Bosch—but members of “The Fusion Project,” as the new alliance has been dubbed, believe these mega-unions only target parts of solutions. Its vision is that industry collaborations should define and activate an entire machine learning data management lifecycle. Specifically, that means tackling fragmented data management, inaccuracies caused by static machine learning models, limited intelligent edge computing capabilities and insufficient in-vehicle computing power.
“Typically, when you see a collaboration like this it’s between two companies, maybe three,” Keefe Leung, Airbiquity’s vice president of project management, said in a video release. “In this case there are five companies bringing their respective products together to show a reference level of integration of automotive-grade software, platforms and hardware. And everything works together to demonstrate end-to-end, upgradeable data collection and edge analytics in the vehicle.”
Users can train specific AI models using the system’s application protocol interface (API). The group chose lane change detection for its debut use case because that capability is a necessary first step to autonomous driving and comprises all aspects of the corresponding data lifecycle.
How It Works
First, vehicle sensor data is collected and processed onboard the vehicle by a combination of NXP’s BlueBox Autonomous Driving platform and S32G GoldBox Service-Oriented Gateway (SoG) platforms, running Wind River’s Linux Operating System and Teraki’s Edge Analytics software.
The customer configures the Teraki edge software to select which driving events the Cloudera ML Platform should ingest. Then, the processed vehicle data is transmitted off-vehicle to the Cloudera Data Platform, which is integrated with the Teraki platform for additional analytics, machine learning, reporting and storage.
Onboard Teraki Edge Analytics Modules are automatically updated by the Airbiquity OTAmatic Software Update Client on the NXP S32G, using Wind River Linux and managed with the OTAmatic Software Update. This enables the machine learning models to retrain and update themselves as more sensor data is collected in different driving situations.
The group claims that its first lane-change AI algorithm initially achieved 90% to 95% accuracy, with the AI model trained up to 98% accuracy after cycling through the platform. These early results, project leaders say, are more than experimental data.
“OEMs can now take that accuracy up to 99 percent plus by continuously training and using a set of sensors they prefer,” Teraki CEO and Cofounder Daniel Richart told EE Times. “They can apply this for any models they want to try. We’ve just made it feasible for OEMs to do the AI training quickly, while they can also use it and implement it on production-grade hardware, not on specific expensive lab cars.”