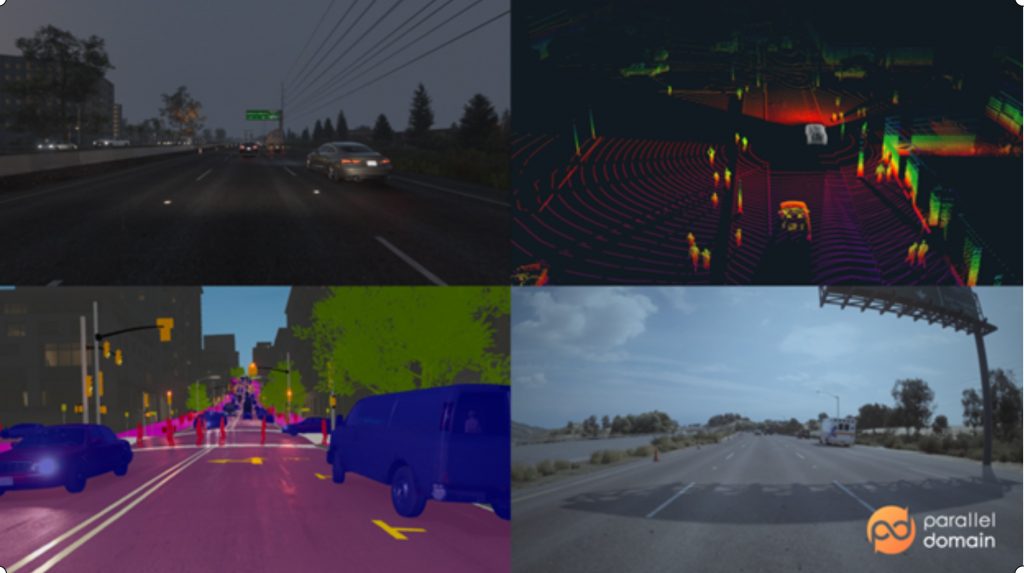
Parallel Domain, a leading developer of synthetic data generation platforms for computer vision development, has raised $30 million in Series B funding led by March Capital, with participation from return investors Costanoa Ventures, Foundry Group, Calibrate Ventures, and Ubiquity Ventures. The company is on a mission to reimagine how AI (artificial intelligence) learns, realizing an autonomous future for everyone and everything with synthetic data.
“We envision a future with accident-free streets, safer skies, smarter homes, and untethered mobility for everyone,” wrote Kevin McNamara, Founder & CEO in a blog post. “When we started Parallel Domain in 2017, we saw that AI models were starved of the data they need to make this future a reality. Five years later, we are delighted that our synthetic data is driving real-world impact for perception teams worldwide.”
Synthetic data will play a critical role in the future of machine learning (ML), added Julia Klein, Partner at March Capital, which brings deep experience scaling growth-stage companies across enterprise AI. Klein is joining Parallel Domain’s board of directors.
“Parallel Domain has emerged as a leader in synthetic data for computer vision, providing tremendous value to the largest players in the fields of mobility, autonomous vehicles, and mobile vision,” she said. “We look forward to working closely with Parallel Domain as they build the elastic compute cloud for data.”
The company’s technology applies to a range of use cases, from augmenting human vision with smartphones to helping drones deliver packages, and building smarter autonomous vehicles. Its growing list of customers, including Google, Continental, Woven Planet, and Toyota Research Institute, are finding synthetic data critical for scaling the AI that powers their vision and perception systems.
“Today, artificial intelligence is stuck making incremental improvements,” elaborated McNamara. “The difficulty of obtaining the right data means that underfed AI systems are struggling to learn at the speed our future demands. This has slowed the development of AI-enabled systems including autonomous vehicles, driver assistance systems, robotics, and autonomous drones.”
According to McNamara, the standard method of collecting and manually labeling data from the real world is prohibitively slow and costly, with developers often waiting weeks or months to obtain new data for improving their models. Human labeling errors, performance issues that result from class imbalance, and restrictions around privacy further hinder ML developers from getting their systems to market.
Parallel Domain’s solution is a platform that enables AI developers to generate synthetic data for training and testing perception models at a scale, speed, and level of control that is impossible with data collected from the real world. The range of data that its users generate in virtual worlds prepares their models for the unpredictability and variety of the physical world.
The value of the company’s synthetic data solution has resulted in significant business momentum, with revenue growing 2.5x year-over-year and its customer base expanding by double digits. Its team has grown to over 80 employees to support customers in North America, Europe, and Asia.
“There’s no other platform for outdoor autonomous systems (vehicles, robots, and drones) today that can generate data at the scale and quality of Parallel Domain,” according to McNamara. “And this is just the beginning. Gartner predicts that, by 2024, 60% of the data used for the development of AI and analytics projects will be synthetic. We are eager to empower every perception team with such capability, enabling a safer and more equitable AI.”
The new funding will enable the company to continue driving revenue growth, expand its team and products to service a broader customer base, and capitalize on the latest advancements in generative AI.
An example of the company’s work was revealed in May 2021 with what it says is the industry’s first public synthetic data visualizer. Historically, high-quality synthetic data has been inaccessible to the general public, but with that release Parallel Domain lets machine learning engineers interact directly with fully labeled synthetic camera and lidar datasets for developing better vision and perception models for autonomy applications.
“With synthetic data, it’s not enough to just have a few great looking screenshots in a limited collection of virtual worlds,” said Wadim Kehl, a Machine Learning Tech Lead at Woven Planet. “You need dynamic scenarios with complex labels and the ability to multiply those things across varied environments & conditions. It’s really great to see Parallel Domain create applications like this that allow the community to experience this caliber of synthetic data to promote better accessibility.”
Parallel Domain’s synthetic data visualizer is free and available for any machine learning engineer or team member at its website. With the ability to visualize synthetic sensor data and a large menu of common computer vision data label formats, machine learning teams can now understand the uses of synthetic data before making decisions on how to best train, test, and deploy computer vision and perception models.
The platform provides two products for generating synthetic data: batch mode and step mode.
Batch mode is designed for machine learning engineers to generate custom datasets to train test or validate their machine learning models. In a single command, users can generate large datasets with specified distributions of weather, scenarios, locations, content, and much more.
Step mode is an API (application programming interface) designed for simulation teams to generate synthetic sensor data on demand for validation purposes. For each timestep, the simulator sends the state of the world to product development’s step API, receiving high-fidelity sensor data corresponding to that timestep in return.
Both modes include high-fidelity annotations that support diverse machine-learning tasks.
All sensor data are captured within virtual worlds procedurally generated from real-world map data. This enables worlds to contain deep synthetic complexity while maintaining realism. As a result, data captured within Parallel Domain’s virtual worlds contain the complex and noisy details needed to accurately model the imperfections of the real world.