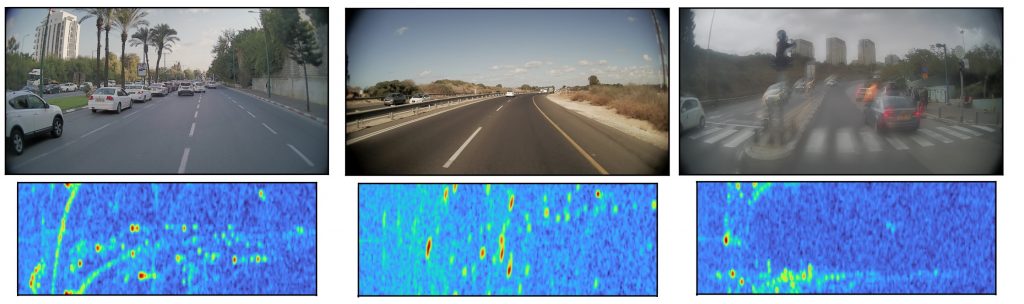
Artificial intelligence technology can quadruple the resolution of radar for use in autonomous vehicles, a new study finds.
Cameras and LiDAR in autonomous vehicles often do not perform well in harsh weather and also suffer from a limited effective range. In contrast, automotive radar is more robust to harsh weather and offers a longer effective range.
However, most commercial automotive radar has poor angular resolution. Angular resolution refers to the minimum distance needed to distinguish between two equal-size objects at the same distance from the observer, which in a vehicle could mean distinguishing between a car driving in a nearby lane and one parked at the same distance along the road. Poor angular resolution hinders driving in environments cluttered with others cars and roadside objects.
One way to improve the angular resolution of automotive radar is by increasing the number of physical radar transmitting and receiving channels. However, this also drives up system complexity, requires sensitive calibration processes, reduces a system’s robustness to hardware malfunctions, decreases power efficiency and raises costs.
Now researchers have developed algorithms that can improve the angular resolution of automotive radar by four times without increasing the number of physical radar channels. The scientists at Bar Ilan University and Wisense Technologies, both in Israel, detailed their findings online Dec. 15 in the journal Science Robotics.
The family of algorithms, named Radar signal Reconstruction using Self Supervision (R2S2), uses artificial intelligence (AI) technology known as deep learning neural networks, which roughly mimic how the human brain processes data. These systems were trained on data collected by synchronized camera and radar systems on vehicles driving through cluttered urban and highway environments in clear and rainy conditions.
The researchers found that R2S2 could fill in the blanks of an automotive radar system’s vision by reconstructing the data that physical radar channels would have received if they were there on the vehicles. They noted that a larger radar array could achieve greater improvements — for example, an array of 64 receiving channels instead of the 16 used in their experiments could result in an eight-fold boost in angular resolution.
“This research paves a new path for automotive radars required for autonomous vehicles,” said study lead author Itai Orr, a doctoral student at Bar Ilan University and chief technology officer at Wisense Technologies. “Current solutions and paradigms are lacking in their ability to answer the needs of high-level autonomy and automotive safety applications. Our approach, which combines deep learning and radar sensing, offers a different way of looking at the problem.”