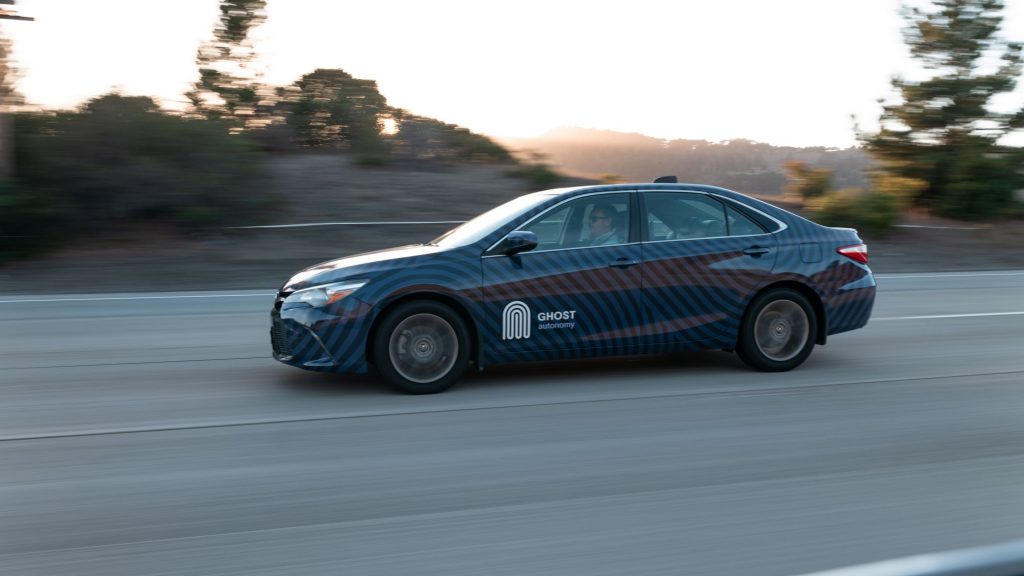
The Center for Automotive Research’s annual Management Briefing Seminars in Traverse City, MI, earlier this month gave a rare glimpse into developments at the relatively unknown autonomous vehicle software startup Ghost Autonomy. CEO John Hayes, who co-founded the company with former CTO Volkmar Uhlig, gave a presentation on his company’s autonomy software for consumer cars featuring new techniques for AI (artificial intelligence) testing and neural network validating to ensure high reliability.
When Ghost started in 2017, Hayes said that his company wanted to go against the dominant autonomy approach with people movers and produce software for autonomy in consumer cars.
“We want to go [into] every car,” he said. “We build on top of ordinary microcontrollers, and not supercomputers in trunks. We focus on making software.”
Ghost uses “late fusion” of vision and radar sensor modalities for “resilient perception,” with a focus on attention-free autonomy on the highway.
“The end experience that we think that everyone is looking for is, you’re driving along the highway, and you just let go, and the car is automated,” said Hayes. “And every car should be automated all the time at a lot of levels. This is [from] the most casual interaction all the way up to an end-to-end, point-to-point navigation system.”
Looking at the California accident reports for AVs (autonomous vehicles), he says that almost all the collisions that occurred were due to software that failed to interpret the environment or had some rigidity that caused it to behave in an unusual way. While the first generation of fully autonomous vehicles focused on AI for perception and then applied it to planning and control, the algorithmic attempts to solve these problems turned out to be unreliable.
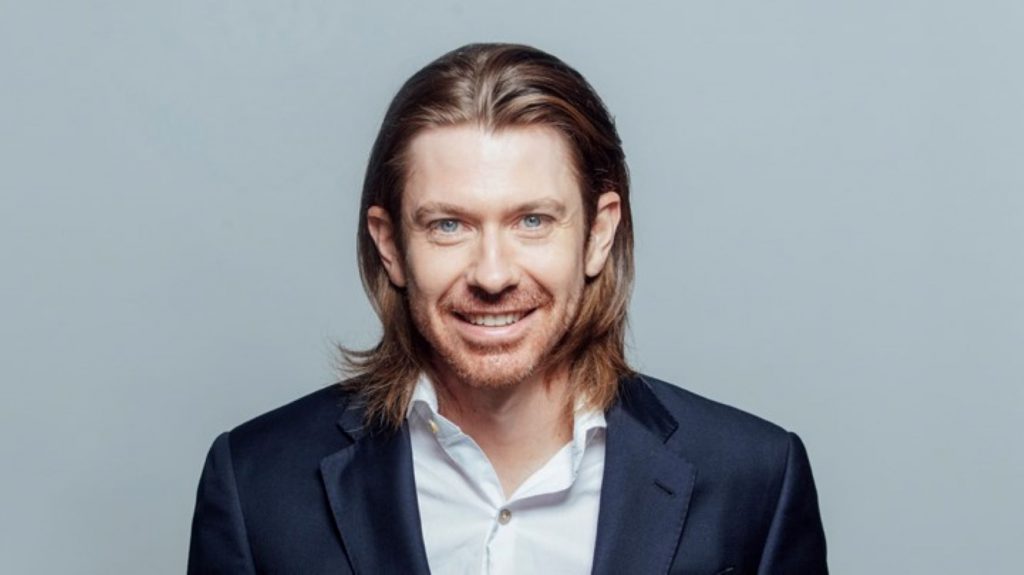
His company proposes a big change in the software development process with generalization as a technology choice. The Ghost Autonomy Engine is being developed to generalize with universal AI, no HD maps, and fully auto-labeled training data.
In June, the company released a video showing its system driving autonomously on a road it had never seen before—Interstate 15 from Barstow, CA, to Las Vegas, NV—traveling more than 140 miles with no safety driver interventions. It maintained an average highway cruising speed of 71 mph, with the only stop being at the state line to swap to NV autonomy plates as the law requires.
Ghost developers believe that many of the typical technology choices—HD maps, image-based obstacle and road feature detection, and hard-coded driving logic—are enemies of generalization. HD maps are difficult to keep current and become out-of-date and obstacles and road features are and look different in different places. Driving program rules that assume “the way people drive” or “the way humans act” in given places all require that autonomy stacks neural networks be re-trained and logic be re-developed as new locations are added to the driving domain (ODD). Such non-generalizing technology approaches generally frustrate consumers and result in slow, limited rollouts of autonomy. Examples are L2+ systems that only work on certain mapped roads and robotaxis that only operate in certain cities and can’t drive to the airport.
When it was architecting the Ghose Autonomy Engine, the company’s mass-market consumer car approach necessitated a focus on generalization to satisfy people who expect to be able to “drive” their cars wherever they want. While it may be harder to develop a system that generalizes up-front, company developers believe it pays huge dividends in avoiding long-tail problems that slow roll-out and scale over time. They made many technology choices that enabled generalization but emphasized three that made a huge impact.
Following universal AI principles, its core visual perception neural network, KineticFlow, isn’t based on image recognition and doesn’t have to be explicitly trained on obstacles to understand and avoid them. It doesn’t care, for example, if fire trucks look different in Detroit vs. Germany.
The company uses no HD maps. Its system drives first-person in real-time, just like a human does, without the need to have the road pre-mapped. This eliminates challenges with driving in new places without maps or issues when maps become outdated.
Its system uses fully auto-labeled training data. While it is important that Ghost’s lane/scene understanding neural network is trained with sufficient data to represent the diversity of lane marker and lane construction parameters where it drives, its process is highly automated and very scalable because it requires no manual human data labeling.
The company began developing its technology and validated it on closed-track environments, then started driving on the freeways around the Bay Area in 2022. In 2023, it is proving the generalization concept by expanding the operational design domain, which currently includes freeways in CA, NV, MI, and Canada.