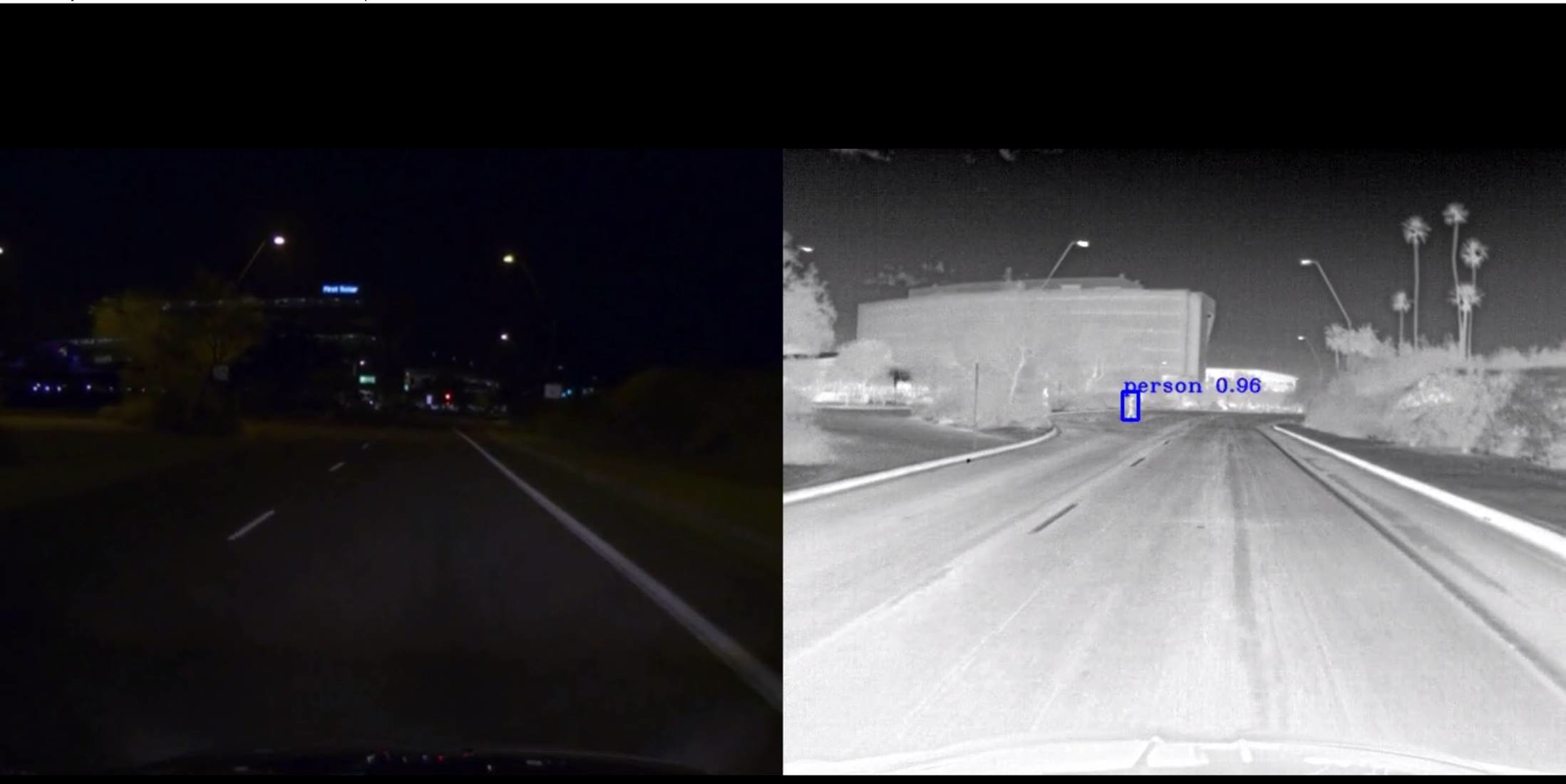
A side-by-side look of the ADK versus a visible camera in challenging lighting conditions at night in clear coniditions. Photo: FLR
When combined with visible light cameras, LiDAR, and radar, thermal sensor data paired with machine learning, helps create a more comprehensive and redundant system for identifying and classifying roadway objects, especially pedestrians and other living things. That’s why FLIR Systems Inc., with over a decade of experience in the automotive industry, wants to continue promoting awareness of the many safety benefits delivered by thermal imaging.
The company has more than 500,000 automotive-qualified FLIR thermal sensors in driver warning systems from automakers such as General Motors, Volkswagen, Audi, BMW, and Mercedes-Benz. Last week FLIR announced the availability of its open-source machine learning thermal dataset for Advanced Driver Assistance Systems (ADAS) and self-driving vehicle researchers, developers, and auto manufacturers, featuring a compilation of more than 10,000 annotated thermal images of day and nighttime scenarios. The first of its kind to include annotations for cars, other vehicles, people, bicycles, and dogs, the starter thermal dataset enables developers to begin testing and evolving convolutional neural networks (CNN) with the FLIR Automotive Development Kit (ADK™).
FLIR is the market leader in thermal imaging products by a wide margin, and wants to grow its technology in the autonomous vehicle market, where it has proven to improve visibility and data acquisition in difficult conditions including fog, nighttime and even bright sunlight.
“We think that volume and that experience through all of the design development and manufacturing work, just puts us in a really great position to bring thermal cameras and add the increased safety to the automotive space,” Mike Walters, FLIR’s VP of micro-camera product development, told Inside GNSS in an interview.
The new thermal dataset — available sometime this month — empowers the automotive community to quickly evaluate thermal sensors on next-generation algorithms. When combined with the other complementary sensors, thermal sensor data paired with machine learning delivers a more comprehensive and redundant system for identifying and classifying roadway objects, especially pedestrians and other living things. FLIR thermal cameras have proven reliable in the classification of pedestrians, bicycles, and vehicles in challenging lighting conditions including total darkness, fog, smoke, shadows, inclement weather, and sun glare at nearly four times the distance of typical headlights.
“This free, open-source dataset is a subset of what FLIR has to offer, and it provides a critical opportunity for the automotive community to expand the data set to make ADAS and self-driving cars more capable in various conditions,” said Frank Pennisi, President of the Industrial Business at FLIR. “Furthermore, recent high-profile autonomous-driving related accidents show a clear need for affordable, intelligent thermal sensors. With the potential for millions of autonomous-enabled vehicles, FLIR thermal sensor costs will decrease significantly, which will encourage wide-scale adoption and ultimately enable safer autonomous vehicles.”
Walters adds that thermal cameras allow drivers to see beyond the headlights, and that the technology has been proven to better identify animals and other objects along the road at night.
It automatically identifies a deer and pedestrians and can generate some type of audio alert to avert hazards.
“Some (systems) deployed in Europe will actually direct the headlights toward the object that it’s detecting and done in such a way that if there’s a person on the side of the road walking toward the vehicle, if this spotlight was directed at the person it wouldn’t blind them. They wouldn’t even know that the cars’ headlights are directed to them. That’s a pretty cool capability,” Walters said.
FLIR sees the importance of safety systems in cars in the advanced driverless systems, as they migrate up into Level 3 and 4, and “We see a bigger role for thermal cameras. We think they are a big part of the sensor suite that’s needed in autonomous driving,” Walters added.
That was the thinking behind the dataset, which enables researchers and developers to accelerate testing of thermal sensors on self-driving systems.
“We basically saw what the role machine learning was playing in the autonomous vehicle space and a lot of that work is being done with visible cameras and visible images,” Walters explained. “We realized that not that many were working with thermal imaging data and that’s what motivated us to release this thermal image dataset.”
While FLIR is known best as a sensor provider, the company “wanted to accelerate efforts and jump start efforts by people” who could take advantage of such an open source system and accelerate testing of thermal sensors on self-driving systems.
“We see that basically every sensor (serves a role),” Walters said. “We don’t see there being a perfect single sensor out there. You will need this fused collection of multiple sensors to provide for autonomous driving.”
He describes the new dataset as more of development tool for researchers and manufacturers to enable them to immediately begin training their neural networks on thermal image data. This latest offering compliments FLIR’s ADK, which is built around the FLIR Boson™ thermal camera core. The FLIR ADK is designed to be a cost-effective way to develop the next generation of automotive thermal vision and ADAS. Thermal
imaging is an optimal sensor technology for pedestrian and animal detection, identifying people in cluttered environments and giving analytics the critical information needed for automated decisions.
To register to receive information about the new dataset, visit www.flir.com/adasdataset